Pushing the boundaries of mobile computer vision
A transformative, multi-level segmentation technology, intelligently processing videos in real-time.
Our technology combines pre-trained and instantaneous machine learning techniques to cut users from their original video background and transport them into a digital domain of their choice.
Segmentive’s state-of-the-art engine intelligently processes videos from the smartphone camera in real-time directly on the device.
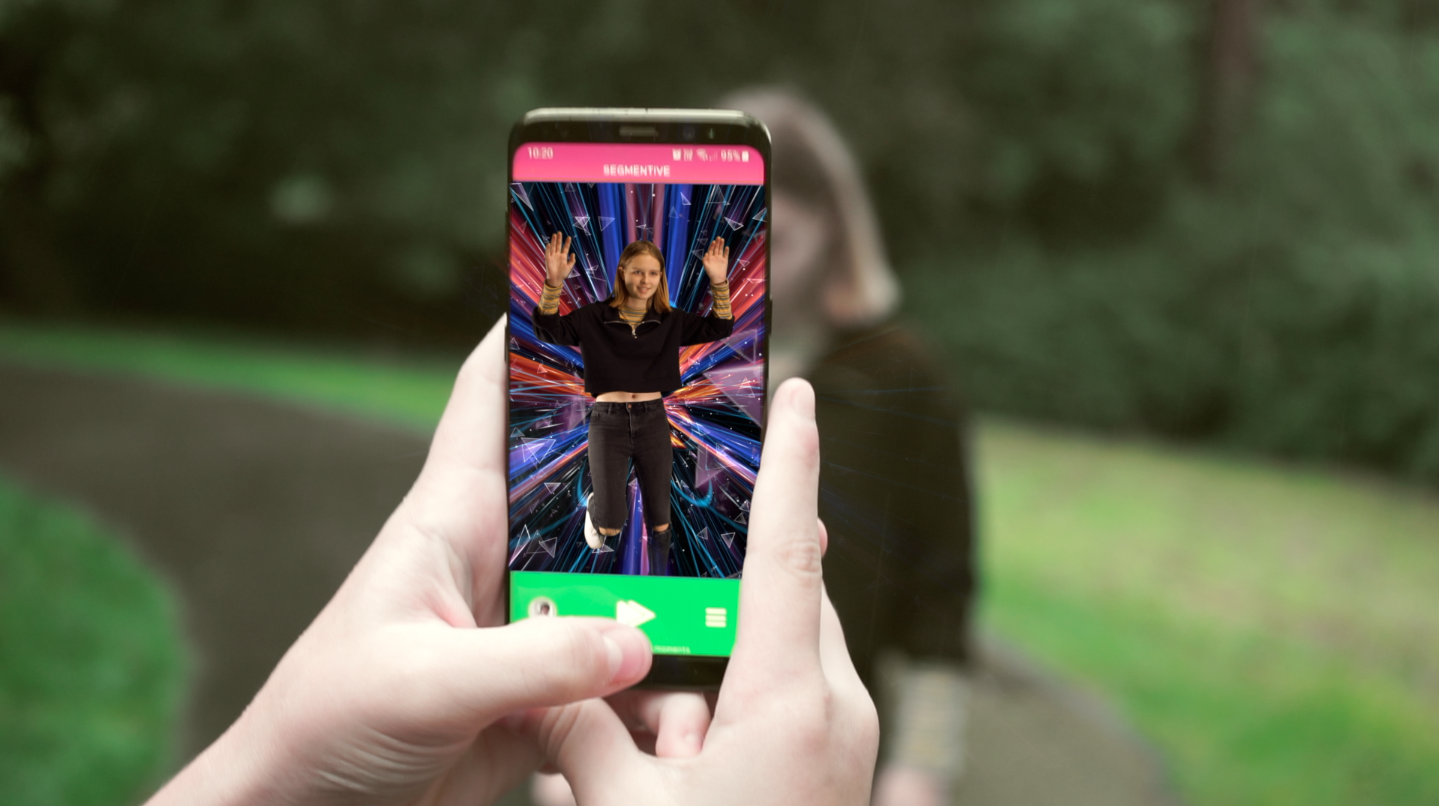
An in-depth look at how our engine works
1 —› Pre-Classification via DNN
A convolutional, 92-layer Deep Neural Network (DNN) is used to pre-classify the frame within the camera’s video data stream into foreground and background pixels. The DNN analyses visual imagery within the frame by using complex mathematical operations that reduce the image resolution and, at the same time, increase the image information available. Segmentive uses its own DNN implementation based on state-of-the-art research approaches. As a result, a 112 x 112 alpha matte is extracted from the video stream.
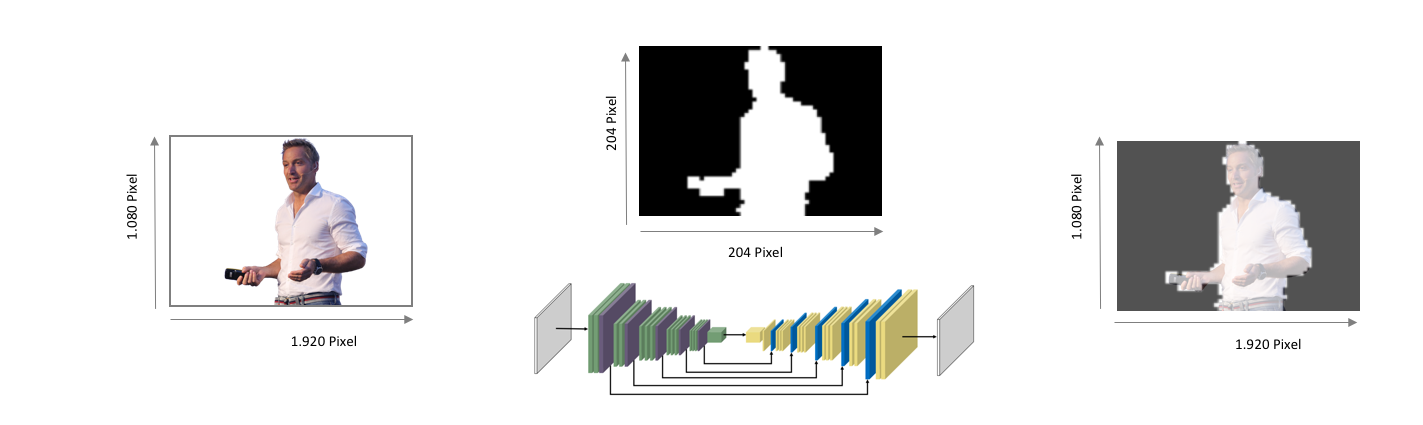
2 —› Fine-Classification via Online Learning Classifiers
During the second stage Segmentive applies a combination of custom online learning classifiers to identify and update the characteristics of the foreground and background of the original frame. These classifiers use the information provided by the DNN as ground truth and learn during runtime. Therefore, they can be kept considerably leaner than the DNN and can classify even fine details on pixel-level in real-time.
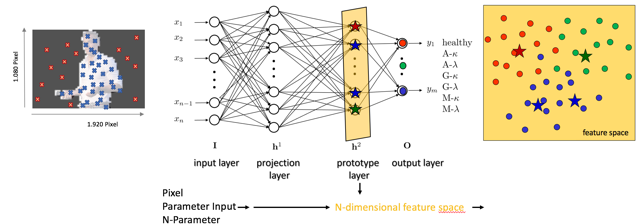
3 —› Third-party data (optional)
Our software is designed in an agile and modular way. As a result, our approach can utilise device-specific information sources (e.g. depth sensors) to generate additional parameters, without the need for pre-training. This data enhances the classification process and the final composition. It also means that our technology can automatically adapt classification to novel situations, such as changes in lighting.
4 —› Final Composition
During the final stage, our software automatically weighs and combines the alpha mattes from the first and second stages. The software splits the frame into different areas based on classification confidence and visual characteristics. This creates a crisp image that intelligently merges the visual details produced by Segmentive’s online learning classifiers pixel-wise classification with the semantic relevance extracted by the DNN.
5 —› The Results
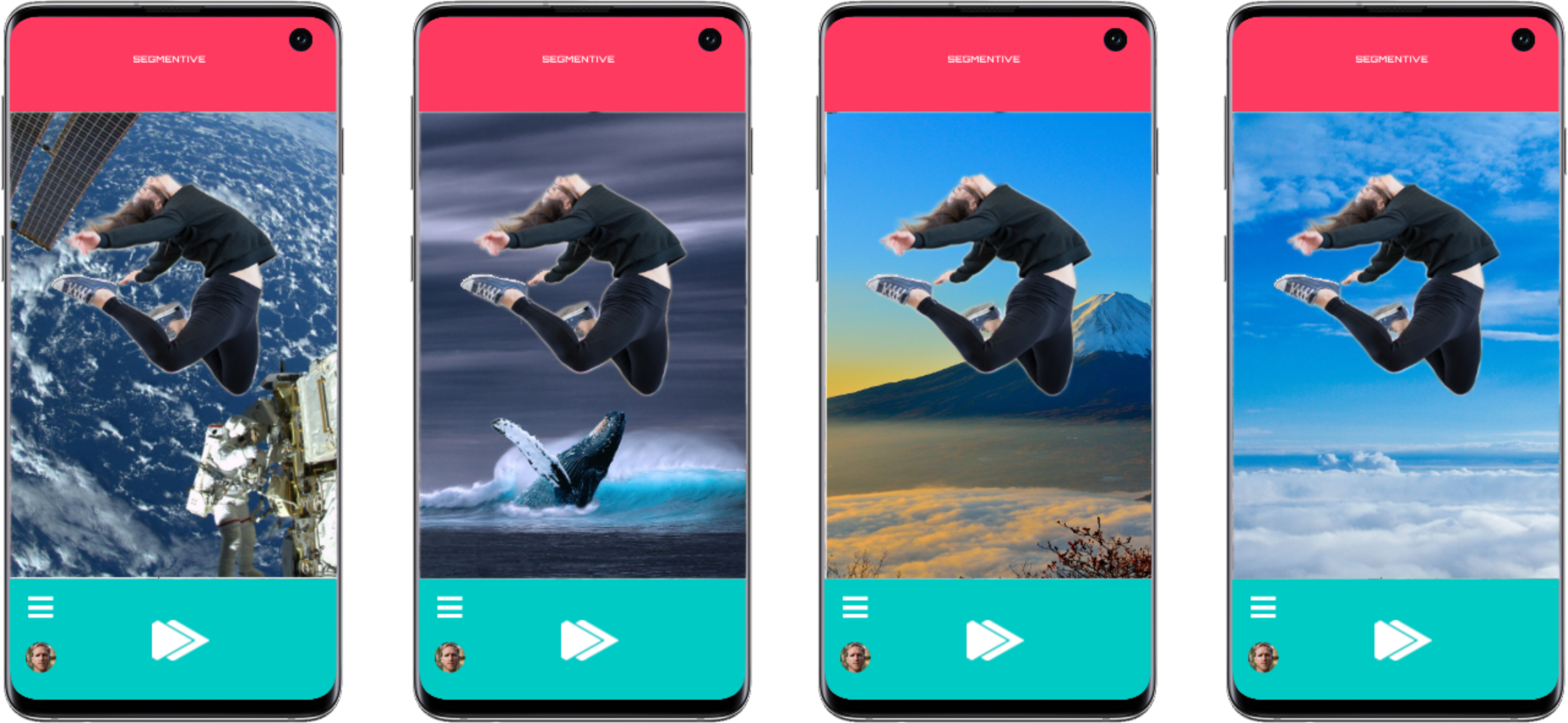
The end result is precise, real-time segmentation of video content, allowing users to be cut from their original video background and transported into a digital domain.